Good morning, friends.
If this is your first time reading my newsletter then I’d like to thank you for swinging by! My newsletter is an attempt to consolidate all of my outputs and Data Science for Arts and Culture is the umbrella term I am using to do that. My outputs can be neatly broken down into three areas: cultural data science; machine learning for creativity and design; and, computational photography — view this diagram here to see more.
Over the last couple of months, I’ve spent a lot of time working on several interesting projects and also jumping on a handful of thought-provoking Zoom calls, which I will link to throughout this newsletter. I also spent some time updating my new website. Please give it a look here to see what I’ve been up to.
Before jumping into this episode, you can read my first episode here and if you think anyone else would enjoy reading my newsletter then please do share with others by simply clicking the button below. It would be greatly appreciated 🙏
What is Cultural data? 🤔
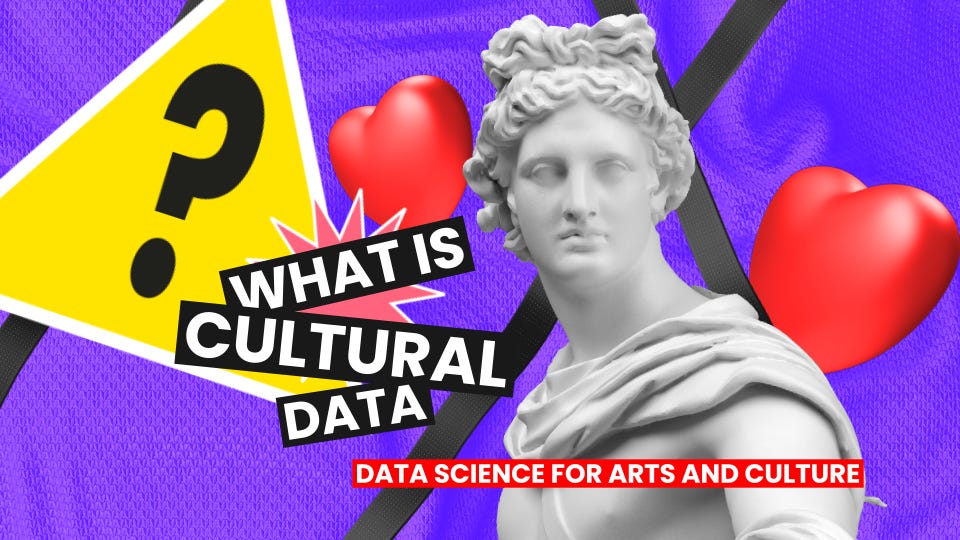
In this episode, I’m going to write about cultural data: what it is and where you can find it. It’s a bit longer than I expected, but if you hang on till the end you’ll see a couple of videos :)
At its most simple, cultural data is described as any data related to art & humanities and culture studies. There are several useful ways to divide and segment cultural data. Cultural data may be qualitative or quantitative in nature and comes in various data types—textual, image and video, sound, numeric, and spatial. Moreover, this data can either be structured or unstructured.
Lev Manovich, the academic who developed the concept of “cultural analytics” in 2005, makes two helpful distinctions in relation to cultural data.
1. Historical cultural data sets 🎨
On one hand, there are data generated within the field of digital humanities. The data generated here is about traditional cultural heritage and artefacts and the digitization of collections in museums and libraries, both visual data and textual data. Manovich describes these as historical cultural data sets.
According to Manovich (2018), the very first project to digitize cultural texts and make them freely available was Project Gutenberg that started in 1970. A few major cultural institutions now have direct access to their digitized collections through an application programming interface (API). The most famous being The Metropolitan Museum of Art, the Natural History Museum, and the Victoria and Albert Museum. Programmable Web has collected access points of over 51 museums here.
Most cultural institutions almost certainly do not offer such access points because a substantial investment is required to do so. Their cultural data sets are probably siloed away on a local server somewhere inside of an Excel spreadsheet. These datasets are private, usually small in size, and have been meticulously crafted and maintained by curators or other museum staff.
2. Contemporary cultural data sets📱
On the other hand, there are data generated within the field of social computing, which is defined by Manovich (2018) as “all computer science research that analyzes content and activity on social networks”. The data generated here is from the “activity on the most popular social networks (Flickr, Instagram, YouTube, Twitter, etc.), user-created content shared on these networks (tweets, images, video, etc.), and also users’ interactions with this content (likes, favorites, reports, comments)”. Manovich describes these as contemporary cultural data sets.
The amount of content being surfaced on social media is astonishing. With over 100 million images and videos uploaded to Instagram, 1 billion hours of video watched on YouTube, and 1 billion videos watched on TikTok every day, our contemporary culture has very much moved online. These data can be mined and analysed to tell us a lot about contemporary cultural trends.
For the last couple of years, I’ve been occupied with analyzing contemporary cultural data sets. For example, I applied natural language processing to 3,000 tweets to understand how people responded to the opening event of the latest London Borough of Culture 2020, and I used computer vision on 9,000 images to understand which were the most popular artworks uploaded online during Frieze London 2018.
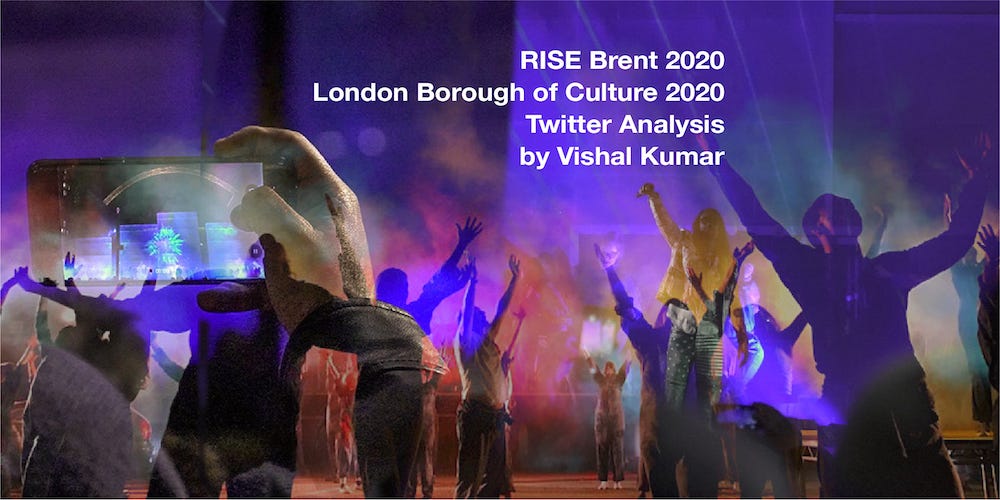
In addition to Manovich’s two distinctions of cultural data, I would include several other types of cultural data sets:
3. Art market data sets 🖼
Art market transaction data is important to consider because it attributes value to cultural data that is able to be traded. Online databases such as Artnet, Artprice and Artory have been recording auction price data for a couple of decades now.
But, one of the biggest challenges with art market data is that almost half of the transactions do not take place at auction. Transactions made by dealers or galleries are done behind closed doors, therefore, no such historical public price record exists for them. Yet, according to Clare McAndrew’s Art Market 2020 report, 40.5 million transactions took place in the art market in 2019… if you compare that to the 100 million images and videos uploaded every day to Instagram that looks puny.
4. Spatial cultural data sets 🌍
Manovich (2018) talks little about cultural data sets that have a spatial dimension. Such data includes the location of cultural buildings, public spaces, or objects on planet Earth using a geographic coordinate system in addition to useful attributes about that building, space, or object.
A relevant repository of such information is the Cultural Infrastructure Map introduced by the Mayor of London in March 2019, which, for the first time, plots and curates the location of cultural infrastructure in London and enables the user to view it alongside other useful spatial contextual data, like transport network data and population growth.
Another example would be point of interest data from Google Places API as part of the Google Maps Platform, Foursquare Places API, or Yelp. Finally, telecoms data and network data such as GPS and WiFi signal data collected at big cultural events, museums, or public areas would be another example of spatial cultural data.
5. Socio-economic cultural data sets 👥
Additionally, socio-economic cultural data sets should also be considered. Arts Council England, a government-funded body dedicated to promoting the performing, visual and literary arts in England, requests a lot of economic data from cultural institutions every year. They then process and curate that information and make it public on their’s or the government’s website.
Moreover, the Cultural Data Project in the US collects detailed information from cultural institutions on revenues and expenses, marketing activities, investments and loans, attendance and pricing, and staffing and volunteers. Furthermore, the European Commission’s Cultural and Creative Cities Monitor is a treasure trove of socio-economic data and indicators to measure the cultural and creative vitality of European cities.
Survey data undertaken by cultural institutions and governments is also an additional source of useful cultural data. The Taking Part survey in the UK is a fantastic example that collects data on engagement in the arts, museums and galleries, archives, libraries, heritage, sport.
6. New forms of cultural data sets 💫
Lastly, it’s worth highlighting the prevalence of a couple of new forms of cultural data.
Open-source urban data from open city portals has also become a source of cultural data. Online platforms such as data.world contains 174 art and culture datasets from mainly North American institutions such as the District of Columbia and the City of New York. Furthermore, the Australian Government has a web portal called Cultural Data Online which provides access to a broad range of research relating to arts and culture in Australia.
3D models and digital art are also new and interesting forms of cultural data. Sketch Fab, a website for 3D models, has a large variety of cultural objects on its website. And, SuperRare, a marketplace for digital art, has a website brimming with cool tradable digital and generative art! These forms for cultural data are likely to become more important as arts and culture moves into virtual and augmented reality.
How COVID-19 has shifted the landscape 🦠
The effects of the COVID-19 lockdowns have meant that many cultural institutions will need to think about shifting some of their cultural data sets online. Dozens of museums around the world offer virtual tours of their museums already, and it’s likely many more will follow suit.
The National Gallery already made a smart investment last year into the “gallery of the future” by collaborating with King’s College London and the Government’s Department of Digital, Culture, Media, and Sport's (DCMS) to create National Gallery X (NGX), an innovation lab within the Culture is Digital policy programme. And, Hauser & Wirth made an investment into their ArtLab and was quick to release VR exhibitions earlier in the year as a response to the lockdown.
Despite the Government’s £1.6 billion rescue package, it’s likely that many cultural institutions might not even exist after all of this has ended. What will then happen to the datasets they own? If they close up shop then the spatial cultural data sets will need to be updated. Joe Dunning from Dunning & Partners writes about the impact of Coronavirus on UK museums here and talks about the importance of partnerships. Whatever the outcome, cultural data sets will need to be maintained regularly over the next decade and this will provide opportunities for those interested in digital and data.
News round-up: What did I get up to last quarter?
As I mentioned in the beginning, I was involved in a handful of Zoom calls during the lockdown. Fortunately, I spoke a lot about cultural data, and I also shared some fairly strong opinions on what I think the future of data is for the art and culture sector. (Hint: it’s not to predict art prices!) Hope you enjoy these :)
Firstly, I spoke alongside Sophie Neuendorf, VP Strategic Partnerships, at artnet, Anders Petterson from ArtTactic, and Giulia Archetti from CADAF about the role of data in the art market. We spoke about the different forms of cultural data, value creation, and machine learning, and AI.
Secondly, I spoke alongside Pontus Silfverstolpe from Barneby’s about the influence of data and analytics on the art market. Some fairly nerdy insights about different analytical techniques were spoken about at length.
What’s next? Cultural data science
In the next episode of my newsletter, I will discuss cultural data science.
Cultural data science can be thought of as an extension of urban studies and cultural studies with a particular focus on gathering cultural data from various sources and applying advanced computational methods to that data to test hypotheses and devise new frameworks with real-world implications to influence policy and market dynamics. I’ll give some practical examples of cultural data science in action next time!
Thank you
Thank you for reading my second newsletter post. Please do consider forwarding this post to your friends or colleagues who might find this content interesting. You can contribute by adding your thoughts in the comment section or feel free to hit reply to let me know what you think. I'd love to hear from you!
Thanks,
Vishal
Vishal is a Cultural Data Scientist & co-Founder of Photogram AI. You can get in touch with him on Twitter or LinkedIn. See more of Vishal’s work on Instagram or on his website.
#culturaldata #digitalart #culturaldatascience
References
Manovich, L., 2018. The science of culture? Social computing, digital humanities and cultural analytics.